ED Data Wrangling Exercises#
Emergency departments around the world must deal with highly attendance numbers on a daily basis. The following exercises work with multiple ED datasets. You will wrangle the dataset into a useful format using pandas
and then visualise the time series using matplotlib
.
The data sets used in these exercises are synthetic, but have been generated to reflect real emergency department demand in the United Kingdom.
Imports#
import pandas as pd
import numpy as np
import matplotlib.pyplot as plt
Datasets#
The dataset syn_ts_ed_long.csv
contains data from 4 emergency departments in 2014. The data are stored in long (sometimes called tidy) format. You are provided with three columns: date
(non unique date time formatted), hosp
(int 1-4) and attends
(int, daily number of attends at hosp \(i\))
The dataset syn_ts_wide.csv
contains the same data in wide format. Each row now represents a unique date and each hospital ED has its own column.
Exercise 1#
Task 1:
Read the two datasets into a pandas dataframe and inspect the columns and data so that you understand the dataset description above.
Hints:
The URL’s for the datasets are provided below.
# your code here ...
LONG_URL = 'https://raw.githubusercontent.com/health-data-science-OR/' \
+ 'hpdm139-datasets/main/syn_ts_ed_long.csv'
WIDE_URL = 'https://raw.githubusercontent.com/health-data-science-OR/' \
+ 'hpdm139-datasets/main/syn_ts_ed_wide.csv'
# example solution
long_df = pd.read_csv(LONG_URL)
long_df.head()
date | hosp | attends | |
---|---|---|---|
0 | 2014-04-01 | 1 | 331 |
1 | 2014-04-02 | 1 | 340 |
2 | 2014-04-03 | 1 | 316 |
3 | 2014-04-04 | 1 | 304 |
4 | 2014-04-05 | 1 | 292 |
wide_df = pd.read_csv(WIDE_URL)
wide_df.head()
date | hosp_1 | hosp_2 | hosp_3 | hosp_4 | |
---|---|---|---|---|---|
0 | 2014-04-01 | 331 | 287 | 195 | 184 |
1 | 2014-04-02 | 340 | 293 | 177 | 181 |
2 | 2014-04-03 | 316 | 249 | 183 | 192 |
3 | 2014-04-04 | 304 | 313 | 208 | 157 |
4 | 2014-04-05 | 292 | 291 | 218 | 166 |
Exercise 2:#
Assume you have only been provided with syn_ts_ed_long.csv
.
Task:
Convert the data into wide format.
the output of your code should a
pd.Dataframe
equivalent tosyn_ts_ed_wide.csv
Make a decision about the appropraite data types for each of the series. For example, by default the attendance column is an
int64
. Is this sensible? What other type of integer could the hospital columns be stored as?
Advanced Task:
Your data wrangling code should make use of chained commands in
pandas
.
Hints
There are various ways to complete this task. You may want to make use of
pivot_table
.One complication with a pivot is that you end up with a
MultiIndex
column for the hospital and number of attends. This is not always particularly clear for labelling. An option is to remove the Mulit-index during wrangling. You could explore the of transposing thepd.Dataframe
using.T
and the.reset_index()
to drop the index.You may want to build up your code command by command to help debug as you go along.
Don’t forget about data types.
# your code here ...
# example solution
def ed_data_to_wide(file_path):
'''
Return the ED data in wide format.
1. Pivot table
2. Transpose and drop the ('attends', hosp_i) multi-index
3. Rename columns [0, 1, 2, 4] tp ['hosp_1', 'hosp_2', 'hosp_3', 'hosp_4']
4. Index to DateTimeIndex
5. Drop the additional uneeded series 'date' (as stored in index as well)
6. Convert attendence numbers from int64 to int16
Params:
------
file_path: str
Path to wide format file
Returns:
-------
pandas.DataFrame
'''
# column name transfers
translated_names = {0:'hosp_1',
1:'hosp_2',
2:'hosp_3',
3:'hosp_4'}
data_types = {'hosp_1':np.int16,
'hosp_2':np.int16,
'hosp_3':np.int16,
'hosp_4':np.int16}
df = (pd.read_csv(file_path)
.pivot_table(values=['attends'], index=['date'], columns=['hosp'])
.T.reset_index(drop=True)
.T.rename(columns=translated_names)
.assign(date=lambda x: pd.to_datetime(x.index))
.set_index('date')
.astype(data_types)
)
return df
wide_df = ed_data_to_wide(LONG_URL)
wide_df.info()
<class 'pandas.core.frame.DataFrame'>
DatetimeIndex: 275 entries, 2014-04-01 to 2014-12-31
Data columns (total 4 columns):
# Column Non-Null Count Dtype
--- ------ -------------- -----
0 hosp_1 275 non-null int16
1 hosp_2 275 non-null int16
2 hosp_3 275 non-null int16
3 hosp_4 275 non-null int16
dtypes: int16(4)
memory usage: 4.3 KB
Exercise 3:#
Now assume that you have been provided with the data in syn_ts_ed_wide.csv
Task:
Convert the dataset from wide format to long (tidy) format.
Advanced task
Your data wrangling code should make use of chained commands in
pandas
.
Hints:
Investigate the
pandas
functionwide_to_long()
or the functionmelt()
# your code here...
def ed_data_to_long(file_path):
'''
Return the ED data in long format. Uses pd.wide_to_long()
Assume wide format file is used.
1. pd.wide_to_long()
2. reset_index() to remove multi-index
3. rename col 'hosp_' to 'attends'
4. date to datetime
5. Convert attendence numbers from int64 to int16 amd hosp_id to int8.
(could also be a categorical field.)
Params:
------
file_path: str
Path to wide format file
Returns:
-------
pandas.DataFrame
'''
translated_names = {'hosp_':'attends'}
data_types = {'hosp': np.int8, 'attends':np.int16}
long_df = (
pd.wide_to_long(pd.read_csv(file_path), stubnames='hosp_',
i=['date'], j='hosp')
.reset_index()
.rename(columns=translated_names)
.assign(date=lambda x: pd.to_datetime(x['date']))
.astype(data_types)
)
return long_df
long_df = ed_data_to_long(WIDE_URL)
long_df.info()
<class 'pandas.core.frame.DataFrame'>
RangeIndex: 1100 entries, 0 to 1099
Data columns (total 3 columns):
# Column Non-Null Count Dtype
--- ------ -------------- -----
0 date 1100 non-null datetime64[ns]
1 hosp 1100 non-null int8
2 attends 1100 non-null int16
dtypes: datetime64[ns](1), int16(1), int8(1)
memory usage: 11.9 KB
Exercise 4#
We will now move onto visualising the dataset using matplotlib
Task:
Using the wide format data, create a line plot of the data for the ED located at hospital 1.
Label the y axis ‘Attendances’
Label x axis ‘Date’
Use a fontsize of 12
Provide a background grid for the plot.
Save the plot as a .png file with dpi of 300.
Hints
Feel free to adapt the plot to improve its appearance using whatever
matplotlib
options you prefer.
# your code here ...
# example solution
fig = plt.figure(figsize=(12,3))
ax = fig.add_subplot()
ax.set_xlabel("Date", fontsize=12)
ax.set_ylabel("Attendances", fontsize=12)
_ = ax.plot(wide_df['hosp_1'], lw=2)
# include x, y grid
_ = ax.grid(ls='--')
# set size of x, y ticks
_ = ax.tick_params(axis='both', labelsize=12)
# save the figure
fig.savefig('hosp_1_ed.png', dpi=300)
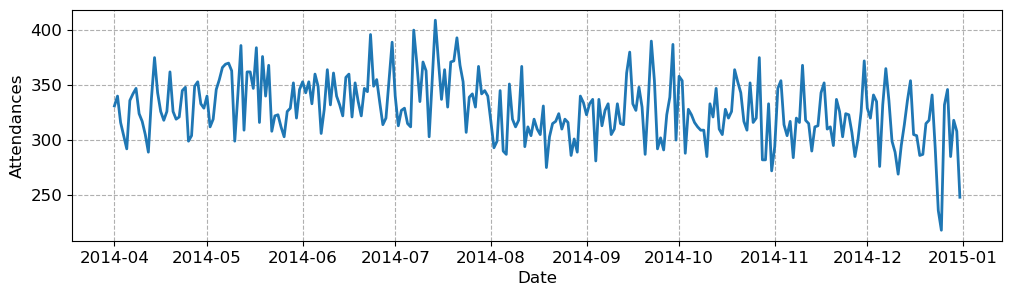
Exercise 5#
Task:
Create a grid of subplots with 1 column and 4 rows. Each subplot should display one of the hospital ED’s.
Label each subplot with the appropraite hospital.
Provide an overall figure y axis label of ‘ED Attendances’
Give the figure and appropriate sizing
Hints:
There are several ways to create a grid of subplots. The easiest for this problem is to use the factory function
plt.subplots()
. Refer back to thematplotlib
sections in the book for help.If you are using
matplotlib
version 3.4 or above you can usefig.supylabel()
andfigsupxlabel()
to set an overall axis label.
# your solution here ...
# example solution
# print(plt.style.available) # list all default styles available
# plt.style.use('ggplot')
# try swapping tight_layout for constrained_layout=True and also omitting it.
fig, axs = plt.subplots(nrows=4, ncols=1, tight_layout=True, figsize=(12,8),
sharex=True)
# note that axs is a 2D array
FS_SUBTITLE = 12
for hosp_idx in range(0, 4):
_ = axs[hosp_idx].plot(wide_df[f'hosp_{hosp_idx+1}'])
_ = axs[hosp_idx].set_title(f'Hospital {hosp_idx+1}', fontsize=FS_SUBTITLE)
_ = axs[hosp_idx].grid(ls='--')
# axis labels matplotlib >=3.4
AXIS_LABEL_SIZE = 12
_ = fig.supylabel('ED Attendances', fontsize=AXIS_LABEL_SIZE)
_ = fig.supxlabel('Date', fontsize=AXIS_LABEL_SIZE)
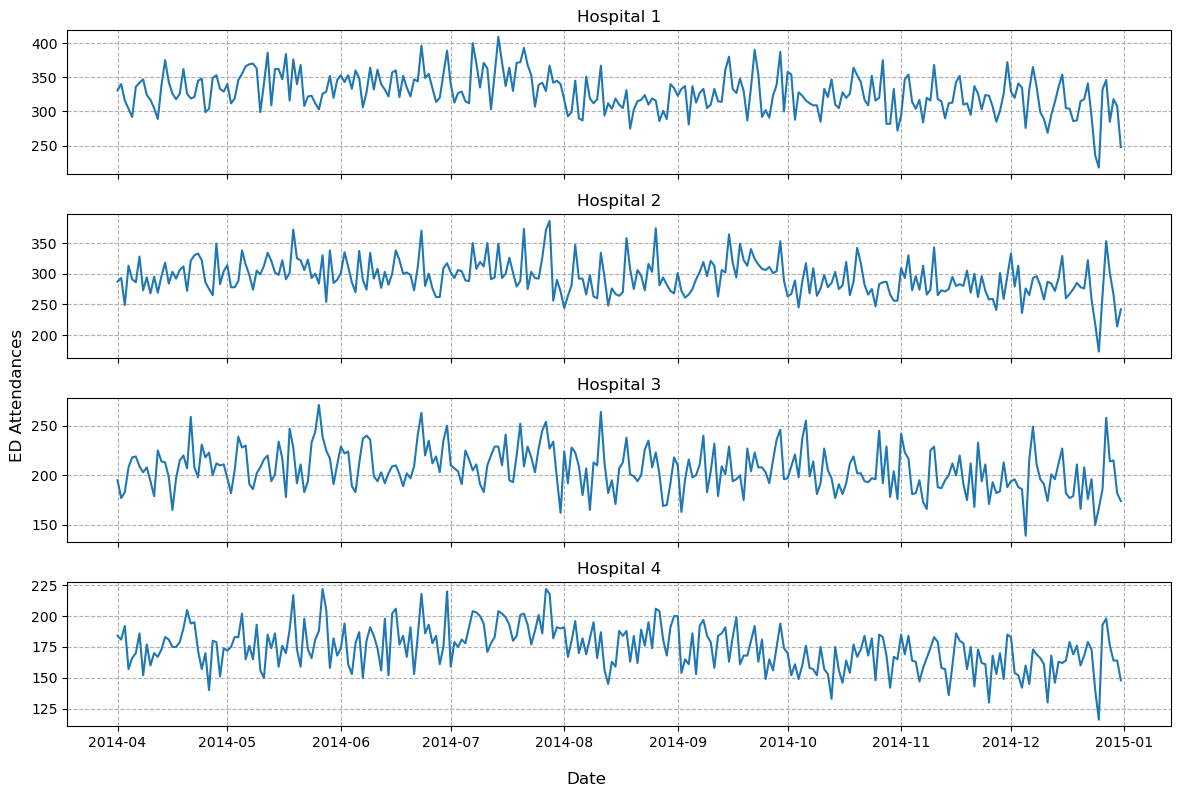